AI and physicians as “thought partners”: a use case in endoscopic colorectal cancer screening
“Integrating AI into clinical practice” has been the holy grail in the field of AI in healthcare. With ML models becoming increasingly ubiquitous and accurate, solving the “integration” problem would substantially move the field forward towards real impact on patient care. But what does “integration” truly entail?
I discuss in this post an example of a recently published AI system for colonic polyp classification that approaches “integration” in what I believe to be a clever way: not just into physician workflow tasks, but also into how the physician thinks. I refer to this concept as “cognitive integration.”
The problem: reduce errors in routine, but high stakes procedures
Colorectal cancer screening using optical colonoscopy (OC) is one of the most effective and highest yield preventative clinical interventions in modern medicine. Unlike most other screening tests, OC can also be therapeutic; if a precancerous lesion such as polyp is identified, it can also be removed during the same session. Therefore, it is critical for polyps to be accurately detected and classified by the physician during a colonoscopy procedure.
For a well trained gastroenterologist, performing a screening colonoscopy is a relatively routine procedure, and some may even think of it as a rote task. However, the stakes are still very high, as a missed polyp can turn into metastatic colon cancer. This combination of high stakes and routine repetition can be a dangerous pair in medicine. Human error and cognitive biases will afflict even the most seasoned physicians, and the relatively routine and repetitive nature of the task may actually amplify some of these biases and potential for error.
An AI system that leverages Bayesian thinking to integrate into physician workflow
Current AI capabilities are still far from being able to completely automate screening colonoscopies, which can often be complex and require human expertise. Rather than attempting full automation, Biffi et al in their article in NPJ Digital Medicine describe an AI enabled computer aided detection (CAD) system that functions as a “thought partner” with a human gastroenterologist to reduce errors in polyp detection and resection. The system, which has two components, functions in the following steps (Figure 1):
A continuous video stream is fed into the CAD system as the endoscopist performs the colonoscopy
The first component of the CAD system initially marks potential areas of interest in the colonic mucosa where there could be a polyp to guide the endoscopist
The endoscopist navigates the scope towards areas of interest (does not necessarily have to be where the CAD system marked) and exposes the colonic musoca to better visualize the polyp
The second component of the CAD system then automatically activates when a polyp is consistently brought into frame by the endoscopist
The CAD system then generates in real time a classification for the polyp in the frame as adenomatous (possible cancer) versus benign, and displays the results of the classification on the screen next to the framed polyp
The CAD system then disengages if the endoscopic resects the polyp or navigates away from it
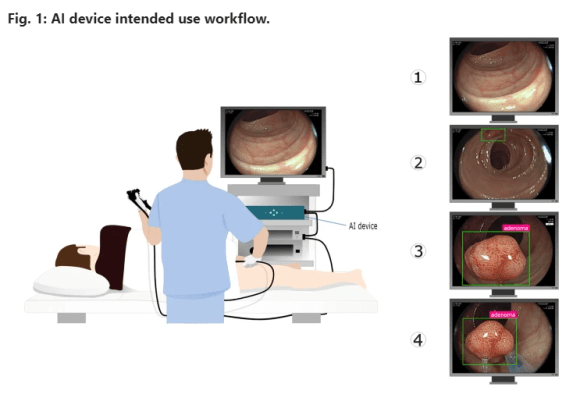
The AI system includes two sets of machine learning (ML) models: the first is a convolutional neural network (CNN) that classifies each detected polyp within a single video frame as “adenoma” or “non-adenoma” and the second is a CNN that generates an image quality score. A key milestone this AI system achieves is the ability to track a polyp across multiple frames in a colonoscopy video and produce a temporally weighted decision (frames that are in focus for longer periods of time are given a higher weight), which is important for real world usability, as images of polyps dynamically change as the endoscopist navigates the scope.
The authors employ a clever strategy of pairing the complementary capabilities and limitations of the AI system and the human physician. The system leverages a Bayesian-guided approach where the AI system, rather than generate predictions independent of any insights that come from the physician and then shows the results to the physician (which is how most AI systems in healthcare operate), actually incorporates prior physician insights into its predictions.
In Bayesian statistics, probabilities are sequentially “updated” with information (priors) to calculate new probabilities that better reflect reality. This is best highlighted in step #4: the CAD system leverages the prior probability generated by the human endoscopist when they navigate to and focus on a suspected lesion, and then deploys the AI classification model on that chosen polyp to generate a conditional probability of whether the lesion is adenomatous or benign. In principle, this conditional probability should more accurately reflect whether a polyp is truly adenomatous than if the AI system naively classified all lesions visualized in the colonoscopy, because it is conditional on the endoscopist focusing on the polyp in the first place, which incorporates their expertise and human instincts.
The study included 513 prospectively acquired polyps from 165 human subjects that were examined histologically to generate ground truth labels. In their primary analysis, the CAD system was non-inferior to expert endoscopists in accurately detecting adenomatous lesions (OR 1.211 [0.766-1.915]) and superior to the accuracy of non-expert endoscopists (OR 1.875 [1.191-2.052]).
“Cognitive integration” of AI into physician workflows
Traditional workflow integration strategies focus on deploying technologies based on how they can streamline physician tasks. However, this task oriented approach misses a critical source of value for AI solutions, which is the integration of AI into how physicians think (and vice versa). This “cognitive integration” will be increasingly relevant as AI systems become more intelligent. The use case described above is a good example of how AI and physicians can team up as “thought partners” to enhance each other’s performance. The CAD system “interprets” the endoscopists’ focus on a lesion as a desire to learn more, and then deploys its AI capabilities on top of insights that the endoscopist already surfaced, which in principle increases its classification accuracy since it incorporates the priors generated by the endoscopist. The endoscopist then uses the AI generated prediction to further hone in on their assessment of the polyp, which also increases their diagnostic accuracy.
When implemented well, this form of cognitive integration between AI and human experts could in theory synergistically enhance the performance of both entities in order to a produce a superior shared result. However, if designed poorly, it could very well amplify biases and lead to poorer performance and other downstream consequences.
The takeaway: when designing AI systems for physicians, consider a cognitive integration strategy that allow the AI and the physician to “make each other smarter.”
References
Biffi, C., Salvagnini, P., Dinh, N.N. et al. A novel AI device for real-time optical characterization of colorectal polyps. npj Digit. Med. 5, 84 (2022). https://doi.org/10.1038/s41746-022-00633-6